Why long samples are one of the keys to improving predictive algorithms in finance
Published 23 November, 2021
Machine learning (ML) algorithms are now ubiquitous in the money management industry. Forecasting the returns of assets is a critical element of building portfolios that perform well “out-of-sample”, in other words, in the future, a period for which no machine learning training data is yet available.
However, there are currently no consistent or established characteristics for asset pricing. As a result, forecasting algorithms can choose from a wide range of parameters.
In a study published in the KeAi The Journal of Finance and Data Science, Guillaume Coqueret, a Professor of Finance and Data Science at France’s EMLYON Business School, considered three dimensions of factor models based on ML: the persistence of the dependent variable, the size of the samples, and the holding period (i.e., the rebalancing frequency of the portfolio). His analysis focused on the US stock market.
According to Prof. Coqueret: “People often seek causal relationships between variables, but they are incredibly hard to reveal and characterise. This paper suggests that finding lasting correlations is roughly good enough.” He adds: “Long-term returns are stable over time and easier to predict, but they suffer from risks of ‘look-ahead’ bias. We cannot use data from the future, so the training samples need to be shifted back in time, which means models may become obsolete. Our study finds that the net effect remains positive.”
He says a counter-intuitive result of this study is that, even if an investor rebalances frequently (e.g., monthly), it is preferable to forecast long-term returns (e.g., annual). “One possible explanation is that short-term returns are very noisy; as a result, models based on them can have a hard time delivering out-of-sample accuracy. It’s as if, paradoxically, the ever-changing short-term relationships are smoothed over in the long term, thereby generating mild inertia,” he adds.
Prof. Coqueret believes his second important finding makes more sense: “Long sample sizes (i.e., deep training samples) are required to optimise the model’s performance. The model needs to learn heuristically from a maximum of data (market configurations) to make the most precise predictions.”
He concludes: “There is now abundant empirical work in asset pricing that relies on big data. A challenging task is to propose theoretical models that can explain the patterns that are observed in markets in a parsimonious manner. This is a central topic in financial economics, and remains more relevant than ever.”
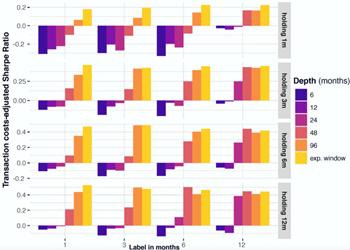
###
Contact the author: Guillaume Coqueret, coqueret@em-lyon.com