Review identifies gaps in our understanding of how ML can aid stock valuation
Published 16 June, 2022
Over the past two decades, researchers have used big data and machine learning (ML) methods to provide insight relevant for equity valuation. Many of these studies either use or inform on accounting variables. In a paper published in KeAi’s The Journal of Finance and Data Science, Doron Nissim, a Professor of Accounting at Columbia Business School in the US, has reviewed a selection of these studies and
identified several crucial areas that have received relatively little research attention to date. In the review, Professor Nissim elaborates on two of these: (1) the use of big data and ML methods to measure intangible assets; and (2) the incorporation of economic, financial and accounting structure in implementing ML algorithms.
In relation to the first point, Prof. Nissim notes: “Organically-developed intangible assets are generally omitted from the balance sheet, resulting in distorted financial statements. This is due primarily to the uncertainty associated with intangibles. Increasing trends over recent decades in intangible intensity and economic volatility have made reported earnings and book value poor proxies for future earnings. For example, research suggests that the underperformance of value factors over the last decade is partially due to accounting measures such as earnings and book value failing to capture increasingly important intangible assets.” He adds: “The dramatic increase in the availability of data and improvements in computing and data analysis methods enables relatively precise measurement of different types of intangible assets. In this review, I look at some recent examples and highlight the need for more research in this area.”
With regards to the integration of domain knowledge and ML, Prof. Nissim believes that big data and ML methods should not substitute for a robust framework that incorporates economic, financial and accounting structure. He explains: “The out-of-sample validity of insights from pure data mining is often questionable. Extracting information from data involves extrapolating from past relationships, but, in some cases, the statement ‘this time it’s different’ actually holds, making that extrapolation inaccurate. Relatedly, judgement often has to be incorporated, which is difficult to do when forecasts and estimates are derived from ‘black boxes’. Simple, traditional models that incorporate economic intuition and provide more visibility into the estimation process are more suitable for incorporating judgement.” He concludes: “The first wave of artificial intelligence models used expert systems, while the second shifted to ML. Combining the two—for example, by integrating domain knowledge and ML—or more generally moving from ‘man vs. machine’ to ‘man plus machine’ approach, may yield superior results. In this review, I provide several examples to support this observation.”
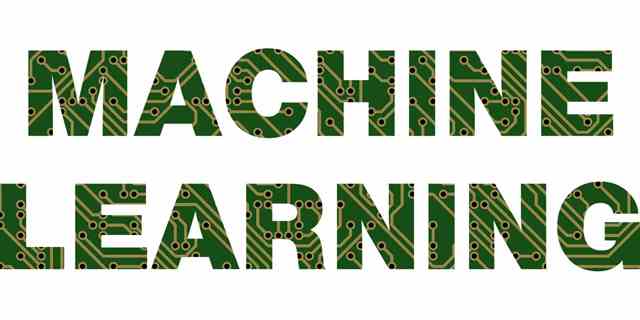
Caption: A recent review has identified gaps in researchers’ understanding of how ML can aid stock valuation. Credit: Gordon Johnson.
###
Contact the author: Doron Nissim, dn75@columbia.edu