DIProT: An Interactive Deep Learning Toolkit for Efficient Protein Design
Published 19 May, 2024
Protein design, a crucial aspect of biological sciences, involves creating amino acid sequences that fold into desired protein structures. This process, known as the protein inverse folding problem, has been a challenge in the field.
To that end, a team of researchers from Tsinghua University (THU) in China introduced DIProT, an interactive protein design toolkit that leverages a non-autoregressive deep generative model to tackle this issue.
"Proteins play a crucial role in numerous biological functions," explains corresponding author of the study Xiaowo Wang, a professor in the Department of Automation at Tsinghua University. "Both predicting the structure of a given protein sequence, as exemplified by AlphaFold, and designing amino acid sequences that conform to a given protein structure pose their unique challenges.”
To developing DIProT, the researchers integrated deep learning models with human expertise directly into the design process, thereby enhancing the efficiency and effectiveness of protein design.
“DIProT's unique approach allows users to specify the target structure and fix parts of the sequence they want to preserve, enhancing the design process's flexibility,” adds Wang. “The toolkit also incorporates a protein structure prediction model to evaluate designs in silico, forming a virtual design loop that significantly improves protein design efficiency.”
One of the key features of DIProT is its user-friendly graphical user interface (GUI), which integrates multiple algorithms to facilitate a fast and intuitive feedback design loop. The GUI allows users to interact with the design outcomes visually, aiding understanding and interpretation of the results.
The authors, who published their study in the KeAi journal Synthetic and Systems Biotechnology, expect DIProT to be highly useful for practical protein design tasks. "We hope that DIProT will stimulate further research in the field and serve as a useful tool for tackling increasingly complex and diverse protein design challenges."
The researchers plan to refine their inverse folding model and toolkit to tackle increasingly complex and diverse protein design challenges in the future.
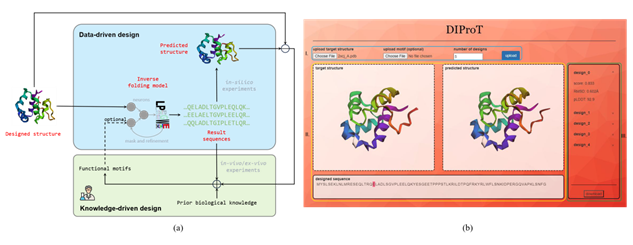
Contact author details:
Xiaowo Wang, Ministry of Education Key Laboratory of Bioinformatics; Center for Synthetic and Systems Biology; Bioinformatics Division, Beijing National Research Center for Information Science and Technology; Department of Automation, Tsinghua University, Beijing, China.
Funder:
This work was supported by the National Natural Science Foundation of China (Nos. 62250007, 62225307, 61721003), and a grant from the Guoqiang Institute, Tsinghua University (2021GQG1023).
Conflict of interest:
The authors declare that they have no known competing financial interests or personal relationships that could have appeared to influence the work reported in this paper.
See the article:
Jieling He, Wenxu Wu, Xiaowo Wang, DIProT: A deep learning based interactive toolkit for efficient and effective Protein design, Synth Syst Biotechnol . 2024 Feb 8;9(2):217-222. doi: 10.1016/j.synbio.2024.01.011.