Enhanced YOLOv7 for Identifying State of Sliding Chair in Railway Turnout
Published 14 November, 2024
The sliding chair is a crucial component in supporting switch rail conversion in the railway turnout. Poor surface conditions of the sliding chair can lead to incomplete conversions. Due to the harsh environmental erosion and the attack from the wheel vibration, the failure rate of the sliding chairs can reach up to 10%.
To that end, a study published in the KeAi journal High-speed Railway introduced a computer vision-based real-time detection method to reliably check the gap state after the turnout conversion by analyzing monitored gap images.
“For the accurate identification of the state of the sliding chair, we proposed an improved YOLOv7-based method,” explains Yuan Cao, first author of the study. “First, the Convolutional Block Attention Module (CBAM) is integrated into the backbone of YOLOv7 to extract more distinguishable features in the images of the sliding chairs, which helps to classify different states with similarities. To detect the sliding chairs with small-scale exposed regions, we further customized an extra detector for small targets into the YOLOv7 network to improve the accuracy of the detection of such sliding chairs.”
For optimal design of the aspect ratio, the researchers revised the localization loss in the objective function, replacing the original Complete IoU with the Efficient IoU (EIoU), which enhances the precise location of sliding chairs.
"Additionally, K-means++ clustering is applied to the anchor boxes in the training set to handle varying-scale sliding chairs, optimizing the priori of the anchor boxes used in YOLOv7,” says Cao.
The improved YOLOv7 is then use to identify the state of sliding chairs. “When deterioration is detected, timely maintenance strategies can be implemented, preventing incomplete turnout conversion caused by degraded sliding chair conditions,” says Cao.
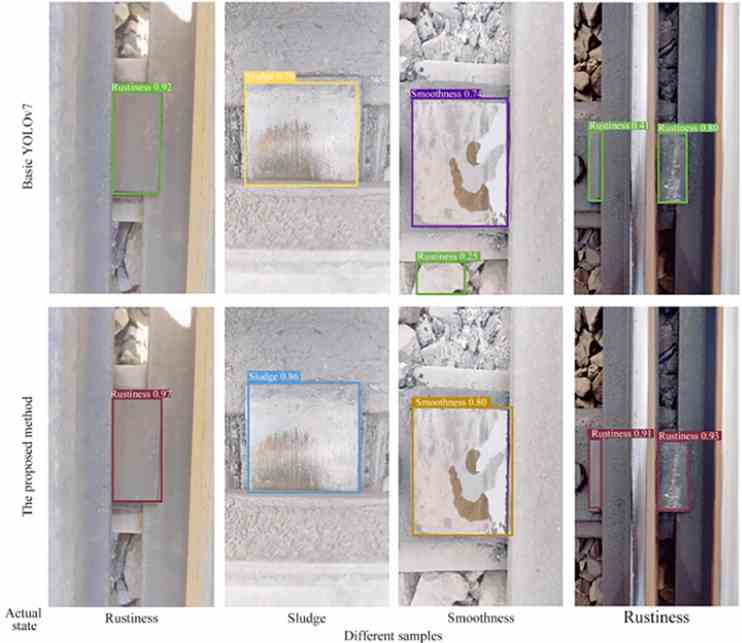
Contact author: Yuan Cao, National Engineering Research Center of Rail Transportation Operation and Control System, Beijing Jiaotong University, Beijing 100044, China, ycao@bjtu.edu.cn.
Funder: This work was supported by the National Key R&D Program of China (2021YFF0501102), the National Natural Science Foundation of China (52372308, U2368202, U1934219, 52202392, 52022010, U22A2046, 52172322, and 62271486).
Conflict of interest: The authors declare that they have no known competing financial interests or personal relationships that could have appeared to influence the work reported in this paper.
See the article: Cao, Y., et al. An improved YOLOv7 for the state identification of sliding chairs in railway turnout, High-speed Railway, Volume 2, Issue 2, 2024, Pages 71-76, https://doi.org/10.1016/j.hspr.2024.04.002