Benchmark Dataset of Seismograms Aims to Improve Earthquake Detection
Published 29 October, 2020
During a natural earthquake, the Earth’s surface shakes due to a sudden release of elastic energy from geological faults. Seismographs record the arrival time of seismic waves generated by this energy release; data that seismologists have drawn on to image and understand the Earth’s interior and dynamics.
Recent advances in both sensor technology and theoretical seismology mean it is now possible to not only leverage seismic signals, but also those connected to ambient noise.
In this paper, authors Fabrizio Magrini et al, make publicly available a global dataset of labeled seismograms generated by both local earthquakes and noise. It is the authors’ belief that local, small-magnitude events, while often problematic to detect, can provide valuable new insights; for example, a better understanding of how earthquakes interact with one another. The paper outlines the application of machine learning to their dataset. It describes how that application achieved accuracy levels of 96.7%, 95.3%, and 93.2% on training, validation, and test set, respectively. It demonstrates that the large variety of geological and tectonic settings covered by their data supports the generalization capabilities of the algorithm, and makes it applicable to real-time detection of local events. It is the authors’ hope that their work will provide the seismological and broader scientific community with a benchmark for time-series that can be used as a testing ground in signal processing.
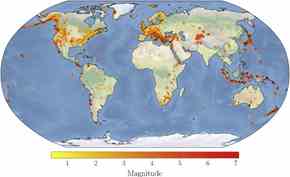
Artificial Intelligence in Geosciences is an open access journal providing an international, interdisciplinary forum where ideas and solutions related to artificial intelligence and its applications in geosciences can be shared and discussed. To support this discussion, we encourage authors to open source their code, data, and the labels used in AI.
Submit today and benefit from:
- Global reach: Papers are hosted on ScienceDirect which has over 17 million monthly users.
- Open access: All articles are made freely and permanently available to readers.
- Article processing charge waived for papers submitted before December 2021.